
MalSnap Project
Cloud computing is one of the most glorified and publicized trends in IT. Most of the organizations today use cloud-computing services (public or private) as part of their infrastructure. Virtualization technology is in the core of cloud computing and virtual resources such as virtual servers are providing services to the whole organization.
​
Due to their importance and prevalence, virtual servers in organizational cloud are constantly targeted by cyber-attackers who try to inject malicious code or malware into the server (e.g., ransomware). Many times, the server administrators do not even know that the server has been compromised, despite the detection solutions that are installed on the server (e.g., anti-virus engine). In other cases, the breach is detected after a long period of time when a significant damage already been done. Thus, detecting that a virtual server has been compromised has a significant importance for the organizational security.
​
Existing security solutions that are installed on the server, detect malware in untrusted manner. Sophisticated malware can evade untrusted detection mechanisms when these mechanisms are observed.
Machine learning methods have been shown to be effective at detecting known and unknown malware in various domains. However, to the best of our knowledge, machine-learning methods have not been used for the detection of compromised virtual machine based on features extracted from the volatile memory dumps taken from the virtual machine.
​
In MalSnap project, we present a novel and trusted methodology for efficient detection of compromised virtual machines on organizational private cloud. We conduct a trusted analysis of volatile memory dumps taken from a virtual machine using Volatility framework. We leverage many features extracted from the memory dump, using advanced machine learning algorithms, in order to better detect malware presence in virtual machine.
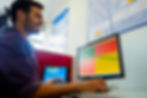

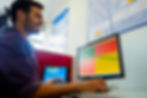